Hand joint coordinates estimation using deep learning on surface electromyography
Summary and data subjects : |
In the research field of active upper limb prosthesis, one of the main issue is the human machine interface. The state of the art is focused for years on a solution to acquire the intention of the subject in order to develop an accurate control of robotic prosthesis. The most promising solution seems to be the processing of electromyographic signals. Suface electromyography (sEMG) electrodes measure a sum of action potentials generated by motor neurons and is widely used in research as it is versatile and non invasive. That’s why various techniques of gesture recognition are developed using surface sEMG. Unfortunately, only a finite number of gesture are classified leading to a small amount of informations to control all the joints of antropomorphic designs of the human hand. In order to improve the motion complexity of robotic prosthesis, this work proposes a database and a signal process to estimate the joint coordinates of the hand from an sEMG signal.
Description : |
The sEMG signal is recorded from a 8 channels myo armband (Thalmic Labs) commercially available. This solution is easier to set than individual electrodes, cheaper and has enough performances for gesture classification. To our knowledge, it is the best choice for a versatile EMG measurement aiming robotic prosthetic control at a reasonable price.
For the kinematic measurement, a Leap Motion is used. This stereoscopic 1.3 megapixels camera extract the euclidean coordinates of each joint of the hand, including the elbow. According to a kinematic model, joint coordinates can be computed from the euclidean coordinates of each joint. The system is interesting for inter-individual measurements as no calibration is needed. Likewise, this system is affordable and versatile.
The database was created recording 12 different subjects (7 males and 5 females) from 22 to 30 years old.
Illustration : |
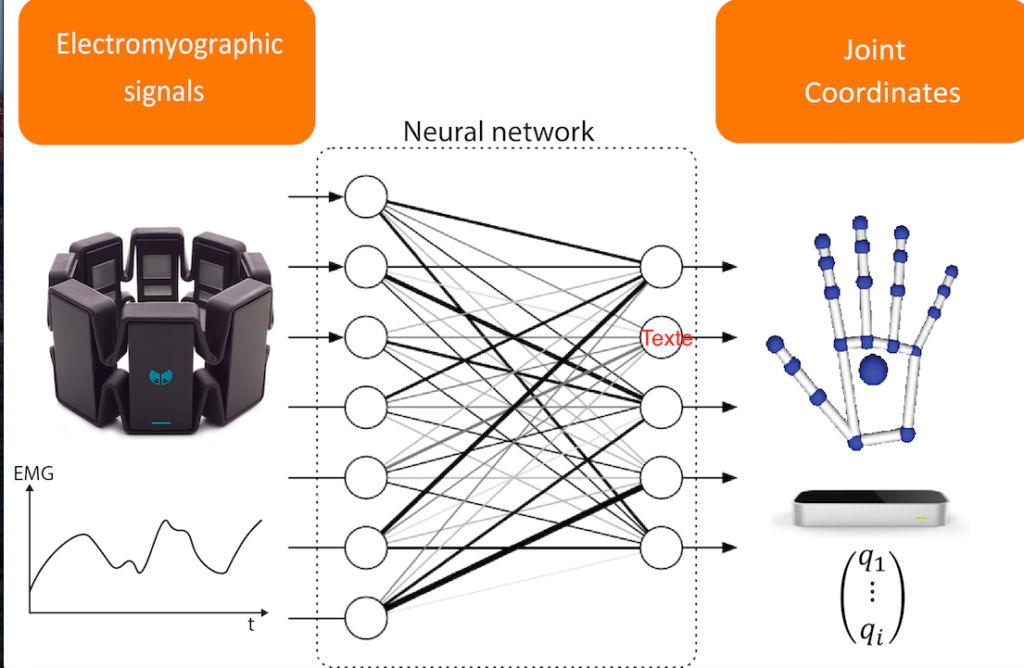
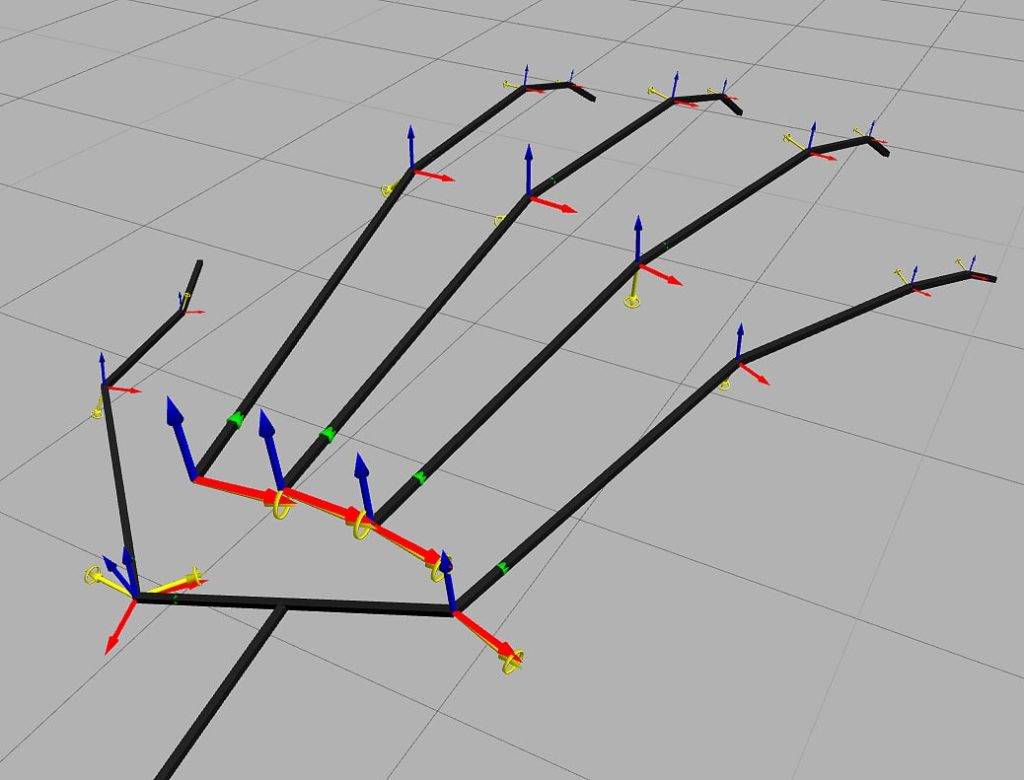